Partnering with AI: Exploring the Potential and Pitfalls of Artificial Intelligence with Peter Voss
“AGI is incredibly valuable— it’s going to be bigger than electricity, and it’s going to be a net positive for humanity.” —Peter Voss
Artificial General Intelligence (AGI) promises to be one of humanity’s greatest achievements. AGI refers to creating computing systems that can learn, understand, and think in the flexible, general manner of human intelligence. If realized responsibly, AGI could help solve our most pressing problems by assisting with complex decision-making, scientific research, education, and more. Achieving this vision of AGI could be a turning point for civilization, but navigating the path will require diligent research, cooperation between experts, and ensuring any advanced AI systems are robustly aligned with human values.
In this episode, JP McAvoy interviews AI pioneer Peter Voss about his journey working on artificial intelligence and his views on the path to achieving Artificial General Intelligence (AGI), a term he coined in 2002. For over 20 years, he has been researching approaches to building artificial general intelligence and aims to develop systems that can learn and reason like humans.
Listen in as JP and Peter discuss the definition of AGI and its potential impact, Issues with current AI models and the need for more efficient approaches, blockchain technology and investment in AI companies, AI’s risks and benefits, how AI can assist humans in decision-making and problem-solving, limitations of current AI techniques, ways for potential improvement, using cognitive AI for customer support applications, and more.
Episode Highlights:
- 03:40 The Need for a More Human-Like Approach to AI Development
- 09:51 Blockchain and Video Tech Investment
- 18:25 AI and Its Potential in Problem-Solving
- 22:40 AI’s Limitations and Potential for Improvement
- 26:14 AI Development with a Focus on Investment and Progess
- 30:53 Cognitive AI for Customer Support
- 37:14 AI and Its Potential to Revolutionize Humanity
Resources:
Get Your Copy of JP’s Book
Quotes:
04:23 “The term AGI is being abused… The term is being hijacked for marketing purposes or money raising.” —Peter Voss
15:09 “AI is one of those topics that people are scared of these days. They talk of AI taking over, they’re an existential threat to humanity.” —JP McAvoy
18:14 “Additional intelligence can help us come up with better solutions. That’s the promise of AGI.” —Peter Voss
30:12 “As the technology changes, the guardrails need to change.” —Peter Voss
39:16 “AGI is incredibly valuable— it’s going to be bigger than electricity, and it’s going to be a net positive for humanity.” —Peter Voss
A Little Bit About Peter:
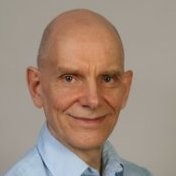
Peter Voss is a visionary entrepreneur with a profound dedication to advancing Artificial General Intelligence (AGI) for over two decades. His relentless pursuit stems from a mission to optimize human flourishing by democratizing access to human-level AI, thus revolutionizing various industries and addressing pressing global challenges.
Leading a major initiative focused on leveraging cutting-edge technology, Peter aims to bridge the gap to wide-ranging human-level capabilities. His journey, from launching successful ventures in electronics and software to conducting extensive research into the nature of intelligence, has culminated in the development of a groundbreaking Integrated Cognitive Architecture, challenging the conventional ‘Big Data’ AI methods. With a commercial product, ‘Aigo – Chatbot with a Brain,’
Peter has set a new standard in automation quality, serving millions and generating substantial revenue. Joining forces with staff, advisors, collaborators, and investors, he invites others to be part of this transformative journey towards human-level AI, shaping the next chapter of human progress.
TRANSCRIPTION:
JP McAvoy: Hi, and thanks for joining us. On today’s show, we’ve got Peter Voss who’s going to discuss all things about AGI, Artificial General Intelligence. Here’s my conversation with Peter Voss.
Hi Peter, thanks for joining us here today, I guess not from sunny Los Angeles. You’re now in Austin, is that right?
Peter Voss: Yes, I moved here a year ago.
JP McAvoy: What inspired you to move?
Peter Voss: Like a lot of other people, I got tired of the California government. It’s so dysfunctional in so many different ways.
JP McAvoy: That’s another podcast with a different subject matter. Obviously for us, we want to talk about AGI. All the discussion around Artificial Intelligence, obviously, it’s a field for which you have a great depth of experience. How did you get it originally involved? How did you really get into it?
Peter Voss: I go back quite a while. So I started off as an electronics engineer, started my own electronics company, then fell in love with software, and my company turned into a software company. So I developed an ERP package for small to medium sized businesses. That company was quite successful. Went from the garage to 400 people, then an IPO. So that was awesome. When I exited their company, it really struck me what big project I wanted to tackle. And the obvious thing was, how can we make software smarter? Software really is pretty dumb. If the programmer didn’t think, or just crash, or give an error message or whatever, how can we build software that can actually have common sense, and that can adapt to changing circumstances not to be brutal. And that led me on a five year research pass where I really wanted to deeply understand all different aspects of intelligence starting with epistemology theory of knowledge.
How do we know anything? What’s reality and philosophy? What’s freewill and consciousness? And then also, what do IQ tests measure? Are they meaningful? How do children learn? How does our intelligence differ from animal intelligence? All of those different topics, I spent five years trying to bring it all together. How can we build computers that can think, learn and reason the way humans do? And so in 2001, I had enough together to actually start an AI company. We started building various prototypes and frameworks. And in 2002, I actually coined the term AGI together with two other people. We wrote a book on the topic of the revival of the original dream of AI from 69 years ago when the term AI was coined. The original dream was to build thinking machines, and they turned out to be, of course, really, really hard. So the field of AI turned into the field of narrow AI solving one particular problem at a time, which is very, very different from building a thinking machine. It’s really the programmers intelligence that figures out how to play chess, or how to do container optimization. So AGI is really to revive the original ambition of AI. And yeah, that’s sort of how I got it going.
JP McAvoy: There’s so many directions I’d love to take the conversation based on what you just said. One of the things that we discuss has been attributed to AGI itself. And that’s just a natural progression, I guess, from what our original thinking of AI was to be to what is coming now. That’s kind of where we’re going with this now, because it’s very topical that we’re going to be talking of AGI. You were doing some of the singing earlier, how have things changed for you? How are people approaching you now given that progression?
Peter Voss: Well, it’s interesting because it’s actually a little frustrating because the term AGI is, again, being abused. And I can give you a very good example. Sam Altman just a few weeks ago said, we are going to have AGI in a year or so. And it’s not going to really be such a big deal. It’s not going to make a big change in the world. Now, that’s nonsense.
JP McAvoy: And that’s not your definition either, is it?
Peter Voss: If he’s talking about AGI, it will make a huge difference, and I believe a very positive one. The term was being hijacked for marketing purposes, or money raising, or whatever. So I’m now starting to talk about real AGI.
JP McAvoy: People say the real whatever, right?
Peter Voss: To get to full human level, the ability to learn like a human, to understand like a human, and generative AI is really not on that path. As amazing as it is, it’s not going to get us to AGI.
JP McAvoy: That’s what he’s speaking of. That’s what they’re trying to accomplish right now. I don’t think we’re not very far away from his vision of what we’re talking about when it’s generative, as you say.
Peter Voss: Generative AI, there are a number of reasons why it’s not on the path to AGI. We’ve gone from the famous paper that launched the sort of GPT, the transformer thing was called as attention as all we need, which was sort of the technical breakthrough to make these large language models work. But now, we can sort of jokingly say $7 trillion is all you need. He’s now talking about building custom dedicated chip factories and nuclear power to power this, and that’s absurd. Our brain uses 20 watts of power or something to do what we do. And here, we have these gigawatts of power for these large language models because they’re just such a brute force approach to trying to get something intelligent, and it’s just the wrong approach. We need something that doesn’t need 100 million, or trillion, or billion dollars to train a model. We need something that can learn more like a child, that can learn interactively stepwise, incrementally, and that will require a tiny fraction of the amount of computing power than these large language models. But that approach is what DARPA calls the third wave of AI, or cognitive AI, and that’s actually the past that we’ve been pursuing.
JP McAvoy: Yeah, that’s what you’re speaking to. So obviously, the topic du jour is these huge models and crunching all data with all kinds of computers. That’s the law, the way that we go to solve technological problems, though, isn’t it? And you’ve done that with others in the past as well. And gradually, things get smaller. So let me actually just ask you in the inverse of what you just said, is it not sort of typical for us to start big, and then Moore’s Law, and continue to reduce to the point where we’re actually able to function with this much smaller, compute much smaller draw?
Peter Voss: I think that’s actually a good question. That yes, we certainly miniaturize things and make them smaller and more efficient. So there is that element to it, but what they’re talking about is bigger and bigger models. Right now, Chat GPT was trained on, I believe 10 trillion pieces of information. And they’re talking about 100 trillion and bigger models. So the models themselves are getting bigger. Whatever efficiency gains, you get by miniaturization. I kind of offset that. It’s fundamentally the wrong approach. The approach starts with, we have a lot of data, we have a lot of computers, that’s a hammer. We’ve got everything that looks like a nail. And as long as I’m making progress with that, it kind of makes sense. But it’s becoming pretty painful. The current models, when companies try to actually do useful things with them, they find that actually very expensive apart from the other shortcomings that they have.
JP McAvoy: What’s produced is not even accurate, right?
Peter Voss: You really want something that can learn more like a child. If they’ve never seen a giraffe before, you can show them one picture of a giraffe and they’ll be able to recognize giraffes from this side, that side of giraffe, and no problem. With statistical models, you probably need to feed them a few thousand images of the giraffe. So it’s fundamentally the brute force and kind of a dumb approach. I mean, extremely effective, but it’s not the way our intelligence works at all. And I believe that is a much smarter way of going about solving AGI.
JP McAvoy: Fascinating to think as it does evolve. The dollars are being thrown, I guess it’s because of the returns that they can see based on approaching in that manner.
Peter Voss: I think we’re seeing a lot of these big bets not working out. The one big company where basically the founders and pretty much everybody migrated to Microsoft, I wonder what’s happening behind the scenes in terms of, that was the company I think that raised $600 million or something, seed round. Who’s ever heard of a seed round of 600, or 600 million, or 400 million or something ridiculous. And I wonder if they still have some of that cash left in the bank. And they say, well, let’s rather distribute it to the stakeholders before it’s all gone.
JP McAvoy: They’ve bailed the investors out. They’ve restructured things, this is what’s occurred. And yeah, as you say that the story hasn’t been completely written on that yet because they’ve repackaged things where they’re used to, at least at this point, not declaring what has been invested all at last because they consider it to be something that’s got this huge potential. I guess that’s what it is, as you discuss this or debate the right way to do it.
Peter Voss: If companies can actually make it.
JP McAvoy: That’s the Holy Grail. It is not to actually achieve whichever way you’re building it. And I certainly appreciate your arguments.
Peter Voss: Well, it depends how much of a moat companies have. Is it going to be the video and the cloud providers that make all the money? And the model builders themselves have become a commodity already. A lot of open source models are coming up and there is a lot of competition. A lot of people are gonna lose money. But I guess that’s what VCs bet on is that they’re also invested in the companies that happen to benefit them.
JP McAvoy: They’re prepared to invest in many of them and will continue to pour into the winners right and not basis. That’s interesting. You say in the video or for open source, and even as you look at blockchain and those that are trying to develop an open source manner that’s traceable through the blockchain, do these projects stand a chance against the behemoth against the ingredients of the world?
Peter Voss: A Blockchain is like a waste of time. I mean, the amount of transactions that you need to do and the cost of a transaction on the blockchain? No.
JP McAvoy: So let me ask you another way, Peter. So as opposed to sort of a blockchain project that is not doing all on chain, I think from what you’re describing there, that’s a non-starter. People are suggesting to sell, to be on chain, but to empower through a blockchain project. So I gotta remember these companies that are emerging, or companies, these initiatives, these data’s emerging, whereby there’s funding through crypto and they’re putting on chain that is required, but coming up with his welder processes so that they’re being owned, not by the large corporations, but by the project themselves. Do you think there’s a future in that?
Peter Voss: I haven’t seen any of those organizations. Kind of makes sense. There’s always somebody pulling the strings and hopefully not pulling the rug, but more often than not pulling the rug. I did spend quite a lot of time in the Blockchain world as well a few years ago. And, just about everything, there’s pump and dump. Apart from the sort of golden, legitimate uses of Cryptocurrencies to be able to move money around the world where you may have government restrictions, of course, a lot of people have made a lot of money just on momentum investing. But that’s speculation.
JP McAvoy: That’s something different. There is money to be made that way. Interesting, though, that you say you spent the time there for a few years ago even those types of uses were considered to be pumping dumps. So it’s interesting how things have evolved to the point where we now consider somebody to be, I don’t know if legitimate is the right word, but certainly here to stay. You see moving funds around or a store of value as we talk about something like Bitcoin. So what was once I think considered to be, well, certainly nefarious is now something that seems to be settled. And so I guess my question would be, can you envision, and I guess I’m asking you to stretch, but I hear you saying, I have not yet seen anything as viable. But can you envision a situation where there is something like that similar product in the future that has the same type of traction, the same type of stickiness? That actually make sense? It’s a very, very broad question. But what are the potentials there?
Peter Voss: Bitcoin is really well established now. So I see that continuing until the government’s outlaws want to compete too much with their own interests. I don’t know where that’s going to where it’s going to lead. But in terms of managing a company, you always have the trust problem that these things don’t solve. And by having contracts embedded, again, it’s something I have experienced. Writing these contracts is also like black magic. And it’s so easy to get the contract wrong, or to have a loophole, or something in there. And again, for somebody to run away, ultimately, it’s not a solid mechanism for being able to trust the system. Trust really needs to be created by a company that you trust the people, the company. That reputation has to be rested with a company. I don’t think you can substitute that by having a blockchain and believing that that takes care of the trust factor. We’ve seen it so many times with these blockchain. Somebody pulled the rug and ran away with the money or whatever, there’s no security in that.
JP McAvoy: It’s interesting that you say that. But the trust of the corporations, I think it’s the current vernacular is the opposite. Then a lot of people listening will say, well, we’re listening and thinking, can we trust the person, the government? Can we trust these corporations? I think that many of them are trying to do the right thing for these reasons. Ultimately, they’re also seeking to profit from them. But it is not necessary, that doesn’t have to go in hand with trying to do the right thing. AI is one of those topics that people are scared of these days. When they talk of AI taking over, an existential threat to humanity. What’s your response to that?
Peter Voss: Yeah, I think it’s very unfortunate that the AI risk community has got so much money over the last 10 years or so. It’s probably a billion dollars by now, maybe many hundreds of millions of dollars, probably more than a billion dollars. And it’s largely driven by people who have a totally theoretical approach to this. And that theoretical approach, I think, is based on some false assumptions. And the problem is, you don’t have anybody on the other side who has any kind of funding, significant funding. You can get funding if you can persuade the world that AI is going to kill us all. But if you give me money, we’ll come up with a solution, or we’ll try and come up with a solution. And that’s sort of being the dynamic. But if you say, no, I think your assumptions are wrong. AI isn’t going to kill us. It’s very hard to get money for that.
JP McAvoy: It’s just not sensational either so you’re not going to get the attention.
Peter Voss: We are all primed with the Hollywood view of AI that it’s always a bad guy and going to kill us and take over the world. And I think they’re just mistaken. I don’t think there’s an existential threat from AI. Certainly, AI will change the dynamic and is changing the dynamic of the world, then we also need to look at the positives. I think there’s a good argument to be made that perhaps we need AGI to save us from ourselves.
JP McAvoy: That’s interesting, because we can’t trust ourselves.
Peter Voss: Well, we need more intelligence. I’m not saying that the AI should be like a parent or the controllers.
JP McAvoy: I think what you’re saying is gonna be a check for what the activity is.
Peter Voss: I think it can make us more rational. Rationality in humans as an evolutionary afterthought, we really are not that good at logical thinking and rational thinking. So if each of us had a little AGI, a personal assistant that we could bounce ideas off of, we would probably make a lot fewer mistakes, and maybe elect better leaders to make better decisions about ourselves, about the environment and everything. That’s so interesting. But of course, AGI has so many major positive benefits to help human flourishing. Imagine just even training one AI, one AGI, or training itself to a large extent up to a PhD level cancer researcher, make a million copies of that, you have a million PhD level cancer research chipping away at the problem. We’re going to make much quicker progress. You take that now to nanotechnology, no battery, energy, pollution, governance, anything where additional intelligence can help us come up with better solutions. That’s the promise of AGI.
JP McAvoy: And it’s fascinating to think where that is going to take us. Can we take advantage of this? I don’t know if, I don’t even know how to choose timelines or time horizons. Correct me if I’m wrong, but the exponential rate of changes as the AI and AGI proved are going to continue to accelerate, if you will, what do things look like in two years now based on what’s currently being developed. What is a day to day life for the average person that’s making use of some of the technologies that are going to be available?
Peter Voss: My own estimate is, as far as generative AI is concerned, statistical AI, I think we’ve probably close to the top of the hype cycle. Companies that have been using this or trying to use and implement this technology for a year are realizing that you cannot rely on it. For example, you always need to have a human in the loop, or you need to put an enormous amount of security around it. Build security around it, and it becomes extremely expensive, and you still don’t have a very reliable system. I don’t see bigger language models and more technology really solving that problem. They’ll become more and more expensive, more and more calm, more sophisticated hallucinations perhaps.
JP McAvoy: You’re saying that that’s not solvable though because it must be.
Peter Voss: No, I don’t believe that with statistical AI. It’s very inherent in technology. There are use cases that we’re already starting to use very effectively such as summarization, idea generation, image generation, many, many things of that nature FAQ the customer is facing. The customer can still see whether they depict the right FAQ or not. But we already had that technology even before GPT.
JP McAvoy: Think we’re Yeah, we’re driving to an answer on it, is because you’re, you’re making references to some of the ways that we’re going to use it, it sounds like you’re thinking that it’s gonna be like an assist to what we’re doing. Yes. So as we continue to use it, yeah, it’s gonna be something we leverage, right? idea generation, for example. Yeah,
Peter Voss: There’ll be a human in the loop at all. We’ve seen it in programming as well. If you’re doing pretty mundane coding and you’re not a very good programmer, then these tools can improve your productivity tremendously. But if you’re doing complex stuff, it is almost completely useless for that. And what I find when I program and I start to use it in something that I don’t, I may not know an algorithm and I’ll try and look it up, it’ll give me the right answer X percent of the time, and they’ll give me the wrong answer sometimes but very confidently. I can’t tell the difference. So I’ll implement the wrong answer, and it won’t work. And then I’ll end up spending more time debugging it. So I’m not finding it super useful in terms of the productivity aid.
JP McAvoy: From what you’re describing, it sounds like you categorize yourself as a superior programmer then, right? I’ve heard people describe how it certainly is, but it’s the assist we just mentioned where an average programmer, or maybe a junior programmer is able to leverage the technology to certainly increase their output or to improve. It’s the super programmers that are saying, people are catching up to us, but they can still feel that upper echelon.
Peter Voss: I think the same is true for an author. If you’re really top class, you write in a certain style. You will notice that a lot of the articles coming out now, obviously GPT style, and it’s a bit painful actually to read them. They sort of have this flowery language and so on. If you’re a good writer, you don’t even want to use it to generate ideas because you want to start with your own outline. You want to have the outline. Now possibly, once you have that, you can throw it into Chat GPT and say, give me some more ideas, and then harvest that. But if you’re not a good writer, then of course, it can help you write something.
JP McAvoy: We’re gonna see a lot more crap today as people are inherently lazy. So only the upper echelon that we mentioned before is going to continue to rise. The vast bulk of it we’re going to speak, continues to be inundated with, unfortunately.
Peter Voss: You actually asked me sort of why I think Chat GPT, one, continues improving to AGI. These problems for hallucination can be solved. And I think you can already see the answer in the name, GPT. G is generative. It’s trained on 10 trillion bits of random information. Not random, but good, bad and ugly data. And it doesn’t know what’s good and what’s bad. There’ll be some clues in there, but it really doesn’t. So it generates an answer. It’s kind of forced to generate an answer when you prompt it with whatever. There isn’t a second level reasoning process, what Daniel Kahneman called the system of thinking. That is sort of the metacognition of monitoring what you’re saying, What are you talking about? Why are you saying this? Or what are the alternatives? They simply don’t have that architecture, and they can’t update their model in real time. So you have a G, generative, that makes up stuff inherently. >You have P, that it’s pre trained, and they basically have a read only model once you deploy them. So as they get new information, or they cannot absorb new insights and update the model in real time. So that’s not how intelligence works. For example, I have a Tesla, and I love the Tesla. But to self drive? It’s not adaptive.
If a particular route, for example, as a human, there might be a new route that you take, and you have to kind of, okay, where do we have to pay attention to get around the corner? There’s a driveway, you already know that. Now, once you’ve driven past there a few times, you are more confident in how you can drive or how you should navigate a particular area, or other clues that you see. You see you hear a siren, or whatever it might be, and these systems are not adaptive. They cannot update the model in real time. And you have the same problem, whether it’s customer service or whatever. You have to retrain the model for anything. So that’s the P, the pre train, which is an inherent limitation. And the T is just the technology, the transformer technology that is used and that really is both the breakthrough in making the system so powerful that the transformer’s attention is all you need. The paper that was published was to announce transformer technology, but that commits you to this backpropagation bulk training that it becomes a read only system. So anything based on transformers cannot be learned in real time. These are not going to be overcome, they’re not going to be little fixes to the system. It really needs a fundamentally different approach. And pretty much, all the luminaries in the field of generative AI, say that we need something else to get to AGI, it’s only when you listen to some old man trying to raise money or as a marketing thing, we’ll have AGI next year. Or Elon Musk saying that we’ll have full driving next year. Yeah, I’ve been driving his car for six years. And if anything, full self driving has gotten worse as it got more scary. I guess that as he gets lawsuits and things, you have to kind of make the thing more and more nervous.
JP McAvoy: I understand the limits of the model. As you described, theoretically, you’re saying it’s not within such a mall. But theoretically, it is possible, right?
Peter Voss: It needs a different architecture. DARPA published something a few years ago, they called Three Waves of AI. And the first wave is good old fashioned AI. What we had in the 80s and 90s, basically logic based systems, expert systems and so on. The second wave as the one we’re writing, we are surfing right now is the big data statistical system. The third wave is a cognitive approach where your starting point is not, how much data do we have? How many computers do we have? What can we do with it? Your starting point is, what does intelligence really require? It requires conceptual understanding, learning in real time, being able to ask questions, and having metacognition knowing what you know. So that’s a cognitive AI approach. But there’s very little money going into that, because momentum is in statistical AI.
JP McAvoy: You can appreciate the money saying it’s easier to build and fund something. But that type of model as opposed to, as you say, cognitive because, where are the goalposts? What are you measuring?
Peter Voss: We have a roadmap with our cognitive AI. Certainly, we could measure that, and it’s not going to be because of training costs. Maybe hundreds of dollars, not hundreds of millions for our system to train. So it’s not that we’re going to need any billions of dollars to make progress on that. Progress can be measured, but investing is very, pretty much a momentum game. And certainly going from Chat GPT 2, to 3, to 4 has been a significant improvement. If you believe that’s going to continue, well, that’s kind of an easy bet in a way.
JP McAvoy: That’s the growth rate and the growth strategy in investing. As you say, momentum is mentioned in trading. Yours, I think suggesting that you maybe want to be betting on another horse. So right or another way, how would you place those bets right now? Especially when you said that it’s not a great deal to actually build it, why isn’t that happening then? And then how do you actually place those bets?
Peter Voss: I’m glad you’re asking. Well, obviously, we believe this is a horse to bet on. They’re just very, very few teams in the world that are actually working on the third wave of cognitive AI, because the oxygen has been sucked out of the air with Gen AI. In fact, we have a whole generation of computer scientists now who don’t know anything other than statistical AI. When you talk about cognition, or metacognition, or concept formation, or any of these sort of psychological terms, they blank out. And unfortunately, VCs get advised by these people. So it’s hard to even kind of anybody be interested in that, because there just isn’t the momentum in it. We tried to educate the public, and we are seeing more and more negative feedback coming out where large corporations are spending a lot of money to try and get Gen AI to do useful things. And they’re finding, well, actually, it’s not that easy to make them work reliably or effectively. They’re also very expensive, and you’re building up this huge technical debt. It was implementing the things that you need to keep tweaking and fixing, protecting yourself and building extra guardrails around. As technology changes, the guardrails need to change. It’s really finding it very difficult technology to actually implement in a practical, useful and cost effective way. So we’re hoping that people will listen more and more to things like we have our technology, for example, it is implemented at one of our customers is 1-800-Flowers, Harry and David Group of Companies, The 12 Companies, and they use it for customer support. A few weeks ago, we had Valentine’s Day. We replaced 3000 agents with our technology without any hallucinations, without needing a separate nuclear power plant to run this. And that is the kind of the promise of cognitive AI where you don’t have a black box you can rely on the results that you can get. It’s just that technology does need to be scaled up to get to human level.
JP McAvoy: I guess there’s one out. You just describe one application, can we go through and speak to that, and in a practical manner. How’s that system been built to serve Valentine’s Day, right? Here’s an example. So Valentine’s Day was serviced. Talk us through what the AI was doing supplementing with the rest of the team was providing to their customers.
Peter Voss: We literally replaced 3000 agents. And in previous years, they had to hire for that. Which, of course, is a big pain. But we provide the service year round. We’re not planning for Mother’s Day, and then it’s over Christmas. It’s just not the numbers that are as high. So basically, the system is deeply integrated into their back end system. So it has all the business rules and has information about that. But the big difference, we call our system a chatbot with a brain because it has short term memory, it has long term memory, it has reasoning ability, it uses context and so on. So if somebody calls in and says, I want to buy chocolates for my niece for her birthday. And as you go through the procedure, you’ll get to know the name. So the system will learn the knees with that name, birthday, that’s a date, like chocolate. So that information will be there and can be used even if it’s not in your back end system. So the system can use information of what you said earlier in a conversation or in prior conversations. We handle replacements, returns, changes to orders, order taking and all of that. You simply could not do that with generative AI. Because if it runs at 95% accuracy and you go through six or seven steps down to 60% accuracy, one in three interactions, you give somebody the wrong price, or wrong return, or whatever it might be. Sell somebody a new Chevrolet truck for $1. That happened.
JP McAvoy: That situation can be quite expensive. So I understand the customer service side of things. And Peter, I’m taking the next thought and hearing so often of people trying to build these personal assistants for individuals, I imagine that similar technology would allow the same thing to occur that way as well. Does that work?
Peter Voss: The beauty of our chatbot with a brain is the brain is really completely agnostic of which application or industry. It basically has conversational ability built in, reasoning ability, memory and all of those things. And then you train it with the additional specific information. Like for 1-800-Flowers, obviously all of our products sort of the ontologies. So if we deployed this for, say a student assistant, it would be hooked up into all of their student systems in the university, whatever their rules are and so on, and then it wouldn’t be a personal assistant. The big difference between cognitive AI approach and statistical, again, the GP that is not pre trained, can learn on the fly in real time. It doesn’t need to be retrained. You simply can’t do that. Well, with generative AI, people try to overcome that limitation of pre-training by external systems. On one hand, you have the input buffer, and you now have some of these new systems like Claude that have enormously big input buffers like 10,000 or 10,000 tokens. But the problem is that information doesn’t actually update the model. It’s just short term memory, and it doesn’t really get integrated. The system doesn’t integrate it into its core model. So that’s, again, a limitation that really cannot be overcome. On the other hand, they use external databases as well to have long term memory. But that again, isn’t integrated and the system has the same kind of accuracy problems in accessing these external databases. So for personal assistance, you ultimately do need to go to a cognitive AI to get really the kind of personal assistant that you want that can learn and have deep understanding about the situation as it unfolds.
JP McAvoy: I hear that, and I agree with you. I’m just wondering, is there a hybrid? I don’t know if that’s even been defined. But is there a hybrid into that history and then also be cognitive?
Peter Voss: Yeah, a lot of people ask you that. It’s kind of an obvious thing to look at, can we not use the power of that. So the only way I see that happening is that a cognitive AI really has to be the main AI that you’re using, and you can certainly use generative AI as a tool. So if I want to write a poem to my girlfriend in the style of, I don’t know, some rapper or whatever, or Shakespeare or whatever, take your pick. And certainly, I wouldn’t expect my AI to do that. It wasn’t trained on 10 trillion bits of information. So you could use it as a tool, the same way that we are using it as a tool. But to integrate them totally and use the power of the large language model constantly isn’t feasible because it’s read only. You cannot update the model. It doesn’t change as your information changes. As a tool, you could use it.
JP McAvoy: Interesting that you say that. Why is that not possible? Why could you take the output and put it into your system and then have it almost be circular to the point where it’s cycled through a number of times before you receive the answer you’re looking for?
Peter Voss: The transformer model basically, really forces you to retrain your whole system. And at the moment for Chat GPT, that’s $100 million. And that is going out.
JP McAvoy: It’s at its limits. From the outset, the model currently exists.
Peter Voss: There’s fine tuning you can do on the model, but that only really changes in the last layer of the model. And if you try to partially retrain a model, it suffers from catastrophic forgetting, which is a problem that’s been long known in the net community. Theoretically, I don’t really see a way of overcoming that. I follow the literature quite a bit on people working on that. How can you incrementally train it? And one of the reasons that it seems impossible is because it is a black box. So the information is so widely distributed amongst its layers, amongst billions of parameters to know which parameters need to be updated if you have a new fact. Just does not seem at all feasible to do that. Whereas with cognitive AI, we don’t have those issues. We just need to scale up the technology that we have. We’re a small company, We only have 30 people in the company. About 12 people are actually in the company now working on moving the technology forward. We’re not going to get to a human level with 12 people all that quickly so we are actually actively looking for funding to expand our team between 50 and 100 people, and then we believe that we can crack this within a few short years.
JP McAvoy: And what type of funding do you require?
Peter Voss: Depending on what milestones you have. Our first milestone is we’re only looking at about 25 million. And then pretty much to get to a point where it becomes extremely obvious that we’ve cracked it, that will be that plus. I just need additional training. We’re looking at maybe 100 million.
JP McAvoy: Nothing like what’s being spent on the other models, but it’s got a keen traction. Away this conversation has been informed, what some of these other early ideas have taken a while to get–
Peter Voss: The issue is, somebody is going to invest in the technology that will get us to AGI, and maybe that’s the person I’m talking to next.
JP McAvoy: That’s right. That’s right. And maybe from this conversation one leads to the other. And the first step is to put it there.
Peter Voss: I’m doing quite a bit of writing. I’m doing loads of podcasts and things to get word out there. AGI is incredibly valuable, it’s going to be bigger than Electricity, and it’s going to be a net positive for humanity. So that’s sort of the first message. Now, not everybody will agree. Some people say, no, I don’t want that kind of world. Or I think it’s going to end in tears. Or I don’t believe it is not inevitable, though.
JP McAvoy: I think that’s it.
Peter Voss: But people may say, I don’t want any part of that.
JP McAvoy: They didn’t want Electricity either. It’s the same type of argument being made. So yeah, I think that for the purpose of this conversation we can include–
Peter Voss: The first thing is, if you believe that, you agree that AGI is something desirable and possible. And then the second thing is to really come to the conclusion that LLM ‘s are not going to get us there. Which as I say, when you talk to the scientists, they say LLM ‘s by themselves are not going to get us there. And that’s pretty consistent. For some of the reasons I’ve mentioned, we need something else. But very few people say what that something else is. And we have an answer to that. We say, we know what something else is. It starts with understanding intelligence, what intelligence requires and building a system that has the capabilities that are needed for intelligence. So that is kind of the conversation I’m having with people, and I’m very much of the mind to help get AGI to happen. I’d obviously like it for our company to do it. But we also are very open to sharing what we’ve learned and what we can do. So it’s really more important for me to have a community that says, we want AGI. Let’s put our heads together and see how we can actually get there. You know, if it’s not not LLM so long, what is it? I believe I have answers.
JP McAvoy: Wonderful stuff. We appreciate you sharing here, and obviously look forward to the community growing as it is. Because as I said, I think that we’re on that path. It’s just a question of when. I think it’s inevitable that we will see that. Peter, what’s the best way for people to reach you if they’re interested in learning more, perhaps, of your company or other things that you’re working on?
Peter Voss: Yeah, my company is aigo.ai, and peter@aigo.ai, or LinkedIn, Twitter. It’s very easy to find me.
JP McAvoy: You’re very easy to spot once you know where to go. So thank you for this. Thanks for being on the show here today. I’d like to ask what was something that people can take with them to the rest of the day, through the rest of the week after the show was dropped. Obviously, we’re discussing some things today that I think are going to impact the future. The way that we’re all doing things, I like the way you describe going back to Electricity.Can you imagine when people were first beginning to look at the adoption of Electricity? What are some of the things people can be doing in their lives now, from your perspective, to prepare for that, put themselves at the forefront of the changes, or put themselves ahead of the curve?
Peter Voss: Clearly, large language models are the tools to know . Being at the forefront, obviously, I would like people to share the word about the third wave of AI, cognitive AI, and also to listen to more people who have a positive view of it and see the benefits of it. And that really, AGI is highly desirable, I believe.
JP McAvoy: I think it absolutely is. I think we will see it here. Thanks so much for joining us here today. I look forward to the next chance we get to chat about the development of AGI.
Peter Voss: Great. Well, thank you, good questions.
Recent Comments